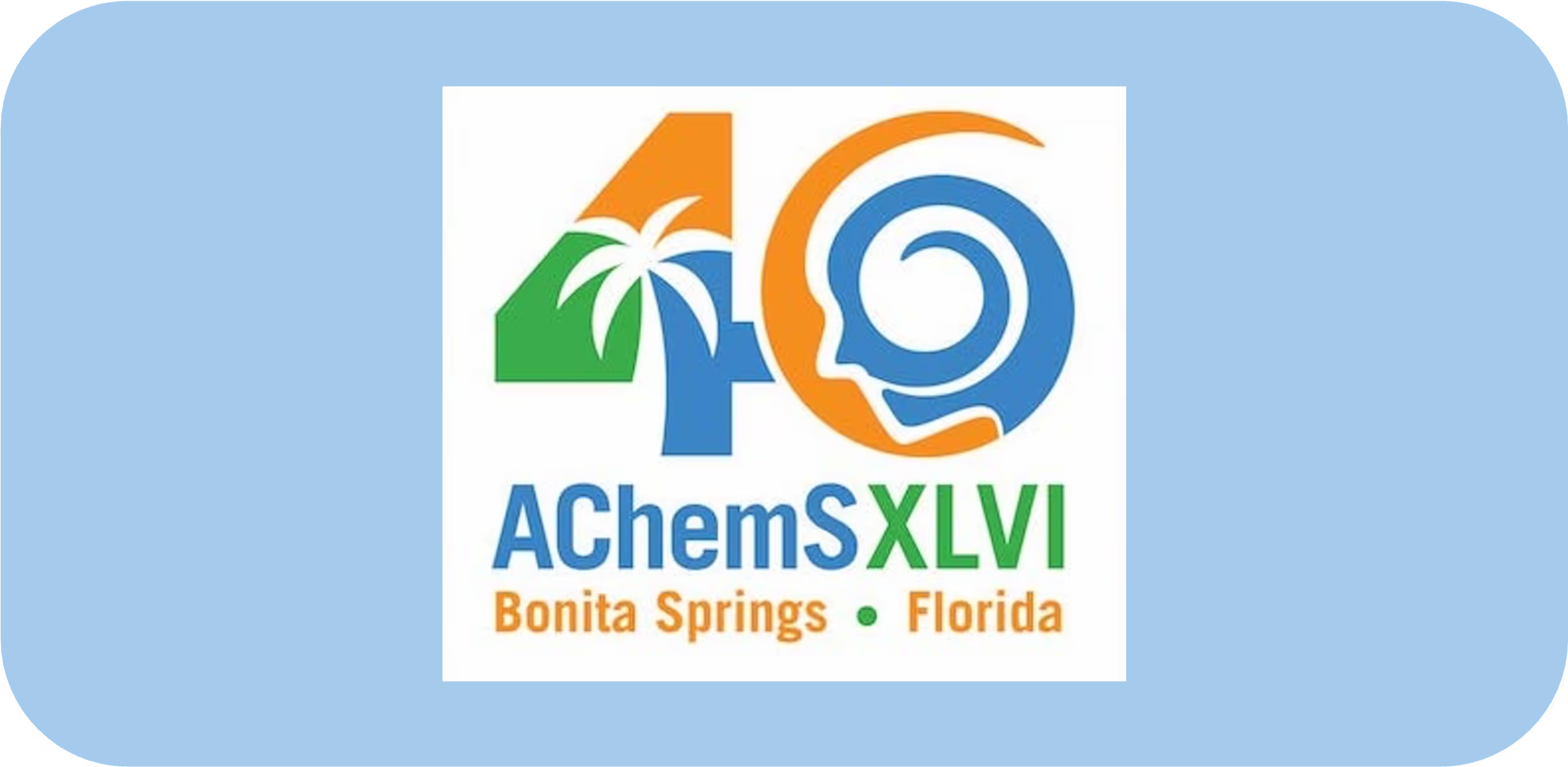
What I Learned at AChemS 2024
Osmo will be the first to give computers a sense of smell and to achieve scent teleportation, but we certainly aren’t the first or only people to work on the science of smell. Prior to joining Osmo, I had attended the annual Association for Chemoreception Sciences (AChemS) meeting a half dozen times as an academic scientist, aiming to share my work and learn about the work of others. Alex and I attended this year to learn about new advances that inform our work and to hear a diverse set of perspectives on what might be required to achieve some of our goals. Here is a slice of what we learned:
Understanding the smell of mixtures might be closer than we think
If you know what all of a mixture's components smell like, can you know what any mixture of them will smell like? There are lots of anecdotal examples of molecular mixtures that don’t quite smell like any of the molecules that compose them. How common is this?
One approach is to create models that predict what mixtures will smell like, using only the data from the components, and then ask how often these model predictions fail. At the meeting it was shown that, in humans, the odor intensity of mixtures of up to 10 components could be well-predicted using only the intensities of the components1. The model underlying this result is neither impenetrable nor obscure. However, quality data collection was key to identifying it.
Another approach is to ask whether the way the brain represents the smell of a mixture (i.e., the pattern of activity of cells in the olfactory regions of the brain) is very different from how it represents the mixture’s components. However, at the meeting it was shown that neural representations of odor mixtures in mice are very similar to what one would predict from the components’ representations2-4. What is the prediction? Simply that the representation of mixture A+B lies on a “path” in neural space between the representations of A and B on their own.
This certainly doesn’t rule out plenty of cases of mixtures that are very hard to understand in terms of their component smells, but it does suggest that these cases are the exceptions rather than the rule. Indeed, entire industries (e.g., fragrance) are based on the premise that a single expert working with a set of ingredients can mix them in ways that are, while not yet perfectly predictable, not completely unknowable either.
By generating large amounts of data, we at Osmo believe we can make odor mixing much more predictable still, by understanding the character of odor mixtures in terms of either the character of the underlying components, or in terms of the chemical structure of the molecules themselves. This unlocks the ability to create any smell on demand, by knowing what components will give rise to a mixture with the intended smell.
Odor is a Low-Dimensional Sense
Color vision is mediated by three kinds of receptors in the eye, and color representation is 3-dimensional (e.g., R, G, B can represent the gamut of color). But there are ~400 kinds of olfactory receptors. So does that mean that odor is 400-dimensional? That kind of simplicity is alluring, but the number of sensors one employs does not imply the dimensionality of their output, much less of subsequent levels of processing that lead to sensory experience. By analogy, the world has nearly 200 countries, but all of them exist on the 2-dimensional surface of a 3-dimensional sphere. Complexity does not imply high dimensionality. Still, odor could be a high dimensional.
At the meeting I saw good evidence that, in mice, neural and behavioral responses to diverse odors can be explained if these odors “live” in low-dimensional spaces akin to a sphere (while a sphere has 3 dimensions, the concept can be generalized to any number of dimensions, the same way that a circle can be generalized to a sphere)5,6. Furthermore, the natural odor statistics of the world around us – the foods we eat and the plants we grow – also seem to have low dimension, possibly best represented by esoteric non-Euclidean geometries and deep mathematical insights, but not an exploding number of dimensions7,8.
The importance of this finding is that low-dimensional spaces are much easier to build predictive models of than high-dimensional ones – partly due to the computational cost of model training, but more importantly due to the cost of the data required to constrain those models. Odor space being low dimensional means that building those models should be within reach for anyone who understands the details of the problem and is appropriately focused and equipped to solve it.
Diverse but Compatible Perspectives on AI and Olfaction
Alex spoke on a panel “The Role of Artificial Intelligence in Olfaction” composed of scientists, engineers, and philosophers from academia and industry9. His position is that AI is a tool that we can use to solve problems outside the capabilities of the unaided human mind, much as a telescope helps you see celestial objects that you wouldn’t be able to see with the naked eye. There was, of course, a lot of enthusiasm and excitement for the potential, but it’s more interesting to distill the skeptical position, which asked the following questions:
- What do you really know if you only understand single molecules in isolation?
- Are odor descriptors enough to understand odor percepts?
- Are model representations related to or aligned with human perceptual representations?
- Is the available data good enough?
- Will any of this understanding be enough to deliver a real odor experience?
I think it is appropriate to bring some humility into our efforts to give computers a sense of smell via AI. This problem is really hard! The approach we cannot take – the approach that led companies down the wrong path with combinatorial chemistry – is that you can take an idea, assume it will automatically work well in isolation, and then walk away. Success requires teams of experts that bridge the gap from AI to the problem domains (chemistry, sensory science, perfumery, etc.) working together to chip away at the parts of the problem that give any one expert the power to do in an hour what it used to take them a week to do. We use AI to help each expert at Osmo work faster, by focusing them on the most promising leads from an otherwise inexhaustible space of possibilities.
As for the other questions, I truly believe we are answering them right now with our current work. That work did not stop when we published in Science10, and indeed our approach has only expanded: our understanding of perception does not end with odor descriptors, and we are always collecting new and complementary kinds of data across a range of techniques and levels of expertise. We are not building opaque models; we are checking “the inside” and consulting with domain experts to make sure the representations produced by our models make sense to humans. And we are going to deliver real olfactory experiences by putting real mixtures of models in front of real people, an activity we already do every day inside Osmo.
We are thrilled to see olfactory science continue to advance in parallel to our own work, giving us independent signals that help us check when we are on the right track, and sometimes creating new opportunities for us to capitalize upon when the time is right. We look forward to demonstrating successful scent teleportation to both the scientific community and the world at large in the coming year!
1. “Predicting Intensity Interactions In Odor Mixtures”, R. Pellegrino et al, Abstract 120, AChemS 2024.
2. “The Order Code”, A. Koulakov, Symposium Session, AChemS 2024
3. “The Geometry And Role Of Sequential Activity In Olfactory Processing And Perceptual Generalization”, Gill et al, Abstract 206, AChemS 2024.
4. “Behaviorally Relevant Features Of The Neural Code In Olfactory Bulb”, Karimimehr et al, Abstract 142, AChemS 2024.
5. “Olfactory Object Representation In The Brain”, D. Rinberg, Symposium Session, AChemS 2024
6. “Glomerular Sequence Represents Odor Quality In The Mouse Olfactory Bulb”, Harvey et al, Abstract 205, AChemS 2024.
7. “Olfaction In Drosophila Through The Lens Of Natural Odors”, E. Hong, Symposium Session, AChemS 2024
8. [Presented but not in conference program], T. Sharpee, Symposium, AChemS 2024
9. “The Great Debate: The role of artificial intelligence in olfaction”, moderated by S. Datta, Panel discussion, AChemS 2024.
10. "A principal odor map unifies diverse tasks in olfactory perception", Lee et al, Science, 2024.
About the Author

Rick Gerkin is the Head of Neuroscience at Osmo. After obtaining a B.S. in Chemistry from Stanford University and Ph.D. in Neurobiology from The University of Pittsburgh, he trained at Carnegie Mellon University and has been a professor at Arizona State University since 2013. He evaluated predictive models of human olfaction at Google Research before joining Osmo at its launch in 2022. His work – combining neuroscience, human psychophysics, and machine learning – has been featured in Science Magazine, the New York Times, the Atlantic, Scientific American, and Wired. For this work, he won the 2023 IFF award in human psychophysics of taste and smell. Rick lives in Phoenix with his wife and three children, and loves Brazilian jiu-jitsu, basketball, and chess.